Abstract:
Nowadays, artificial intelligence is revolutionizing many fields, including mathematics. As an important branch of bioinformatics, phylogenetics, which is the study of evolutionary relationship among species, is a representative example where AI has accelerated the advancement of the field. Traditionally, phylogenetic analysis using protein data relies on amino acid sequences. However, AI-based protein structure prediction tools have enabled structure-based phylogenetics, which is considered more reliable. In this research, we explore the uncertainty in the structure-based method and find that the results are quite interesting.
Blog:
Nowadays, artificial Intelligence is rapidly transforming the world, revolutionizing industries, and reshaping scientific research. In mathematics, especially statistics, AI helps conduct data analysis, improve predictive models, and uncover hidden patterns in large datasets through machine learning and deep learning techniques. Phylogenetics, a branch of bioinformatics, is a prime example of how AI drives the advancement of this field.
In 1859, Charles Darwin introduced the theory of evolution, using a tree metaphor to illustrate the common ancestry and divergence of species. Building on that concept, phylogenetics aims to study the evolutionary relationships among organisms and infer their common ancestors. By using either genetic, protein, or morphological data, it constructs phylogenetic trees to reveal how species have diverged from common ancestors. In this research, we focus on phylogenetics using protein data, and until recently, most phylogenetic analyses (that use protein data) were conducted using protein amino acid data.
In recent years, the emergence of AI-based protein structure prediction tools (e.g., Alphafold 2) has infused new blood into this field. This breakthrough has made it possible to perform structure-based phylogenetic analysis, which is considered more reliable than traditional sequence-based methods, as protein structures typically evolve more slowly than their corresponding amino acid sequences.
In the structure-based phylogenetic analysis method, in order to make use of the protein structure, they utilise a deep learning model called vector quantized variational autoencoder (VQ-VAE) to translate a protein structure to a sequence called 3Di sequence, where the 3Di sequence is made up of letters (they call it 3Di letters) that have limited alphabet size. For such a translation, the downside of it is that since there are almost infinite types of local structures but only 20 3Di letters, we will lose information.
Motivated by this, in this research, we explore the uncertainty in the translation. Specifically, for each position of a protein, we calculate the likelihoods of the position belonging to different 3Di letters. Then, we retrieve the one that corresponds to the 3Di letter of the position to represent the uncertainty of the position. For example, if a position of a protein is translated to “A”, then we use the likelihood of the residue belonging to “A” to represent the uncertainty of the position. Using this information, we perform phylogenetic analyses that incorporate uncertainty information. The result suggests that our method that trims high uncertainty columns in the alignment could help remove columns that are less informative. For future work, there are still lots of things we could do, including improving our method and conducting our method on more datasets.
At the end of this blog post, I want to mention that AI has become an indispensable tool for statistics. And I believe more and more fields of mathematics will benefit from AI in the future.
LI FU ZHANG
The University of Melbourne
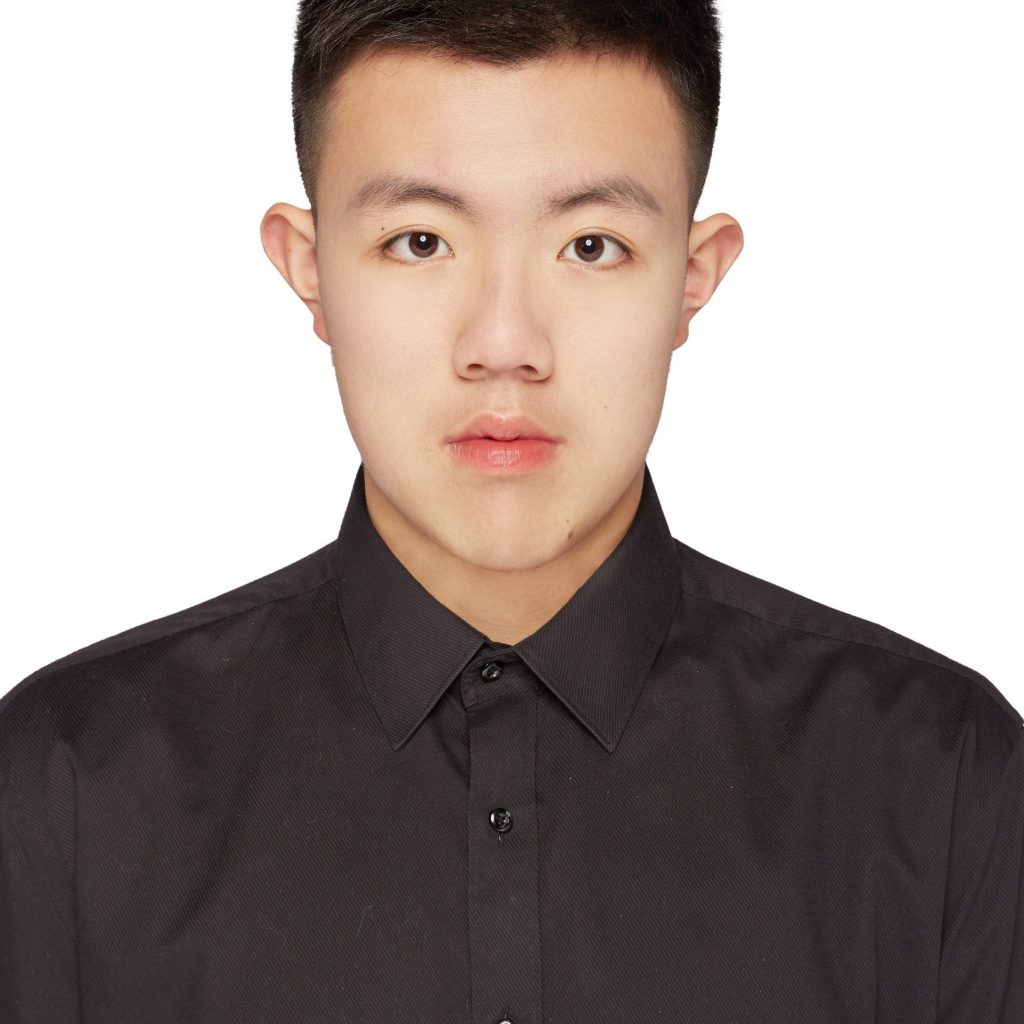