My blog post briefly summarises an interesting example used to teach students about application of conditional probabilities—the high rates of false positives compared to true positives when testing a population for a rare condition. This demonstrates some of the intuition behind Bayesian statistical theory which I applied in my summer project.
A conditional probability is exactly what it sounds like; it’s how likely an event is, given some other event has occurred previously. When you study probability maths, it’s very likely your first examples of conditional probability will be in the setting of diagnostic tests.
Let’s say we have a test that’s pretty accurate—only 1 in 1000 results are incorrect, that means sick people return positive results and healthy people negatives almost all of the time.
If, during routine screening for a particular illness, you received a positive result you would likely become very concerned as we are quite confident that the test works well.
Now consider another key piece of information—the prevalence of what we’re testing. Let’s say only 1 in 10,000 people actually have this disease, so it’s a somewhat rare illness.
Now finally, we can evaluate how concerned we actually should be by combining the pre-existing knowledge (how common the disease is) with the data (our test result).
There are two paths to get to a positive test result:
Option 1 you have the condition and correctly test positive. The probability of having the condition is 1/10,000 and the probability of then testing positive is 999/1000. Combine these by multiplying and we get 999/10,000,000 = 0.0000999, or just under 1 in 10,000.
Option 2 you don’t have the disease (9,999 out of 10,000 people) but the test didn’t work correctly and you return a false positive. Multiply these probabilities to get (9999/10,000)x(1/1000) = 0.00099999, or just under 1 in 1,000.
Now ignoring all the messy 0’s, 9’s and decimal places what’s important is that it’s significantly more likely (roughly 9 times) that you had a false positive result than you’re actually unwell. Of course you’re still going to follow this up with your GP, but hopefully you’re now appropriately concerned as opposed to outright panicking.
This example is a simplification of the real-world scenario—but it represents some of the philosophy behind Bayesian statistics. The idea is that when we look at new information or data we can’t consider it in isolation from the entire body of research that already exists.
This approach is somewhat intuitive, for example my undergrad biology project found sea snails from the temperate Victorian coast are more comfortable in dry 35°C conditions than 25°C conditions. Now upon discovering this we could have madly rushed to get in contact the Journal of Ecology to let them know our current understanding of snail physiology was completely wrong. Or we could do what was actually done, and conclude our data was most likely flawed due to our prior knowledge of the experiment: it was done over merely 3 days and by inexperienced, perhaps even inattentive students.
My research project this summer has utilised Bayesian statistics and although I’m still far off from understanding every aspect of its uses and usefulness—I’ve engaged with these techniques on a small scale to better understand the practical application of this theory. Although Bayesian statistics may seem complex, it stems from an arguably intuitive way of looking at things, and for me, that’s really exciting.
Meg Tully
The University of Melbourne
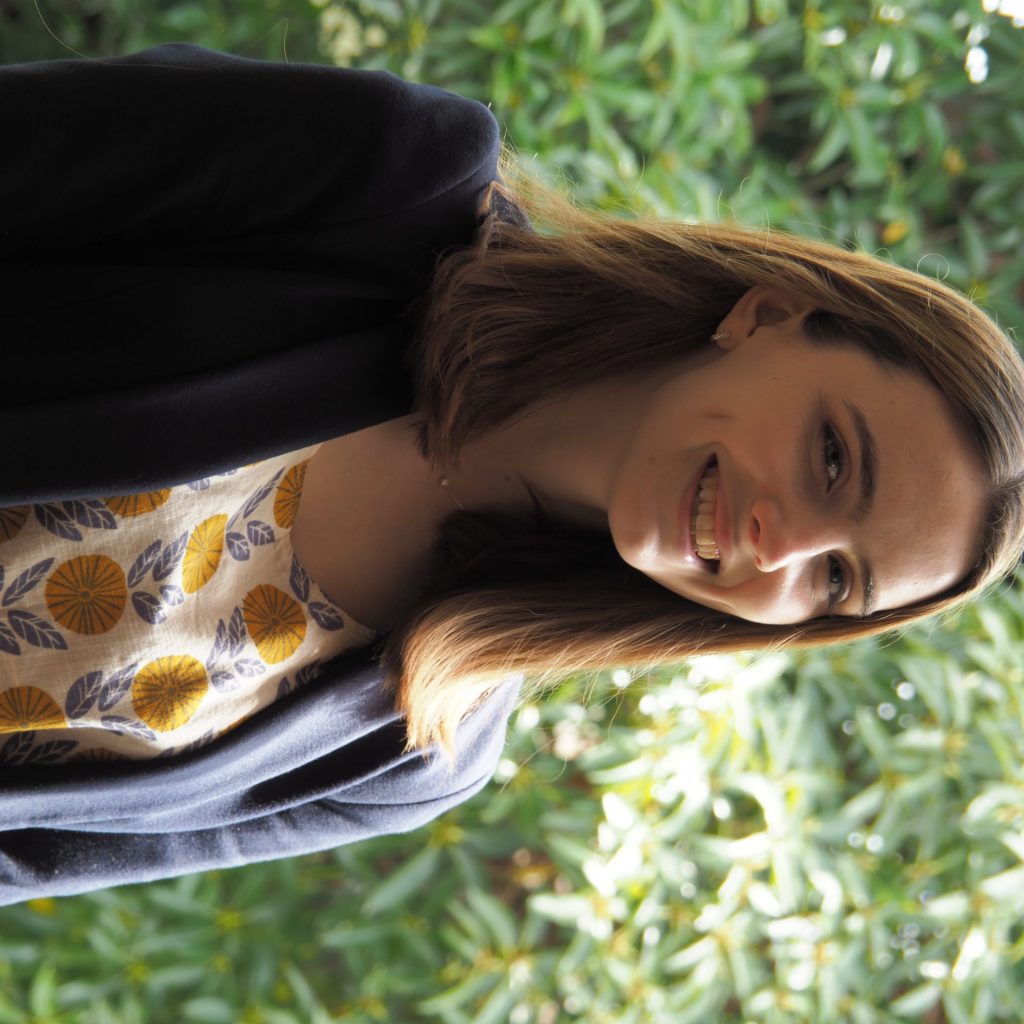