Not sure how are you feeling when you first create a document and plan to type something for your blog? If you come and ask me the same question, I would say I had no clue to write anything at that moment.
If you had the same feeling as mine, I believed that also would be the main reason you saw this blog. I recommend you do the same thing as I did now, start writing something (even meaningless) and continue repeating this step. You might have some ideas coming to your mind then, just like me.
I still remembered how I felt, when I planned to apply for this summer vacation project but found it was going to close soon. With as much courage as I had hesitancy, I finally decided to seek help. Thanks to John for his generous support during those busy days, it definitely calmed my anxiety and raised my expectations and enthusiasm on this project. I would also say my greatest appreciation to John and Sleiman during these six weeks, having the responsible and easy-going supervisors could be one of the most enjoyable things in the research project experiences.
Back to my research topic, it was about statistical modelling. It came from a conversation between John and me.
“Which area are you interested in?”
“Ummm, not sure honestly. Perhaps modelling?”
I was a little unsure on my research topic at that time, but we finally decided it fortunately, which was modelling the climate time series. It was not my first time to encounter a modelling problem with time series data, but it definitely was a brand-new experience for me to study time series models deeper than before.
Researching on the terminologies and what others did on this topic, studying on the time series models, asking the questions, writing the codes, experimenting the models, encountering the problems, seeking help, fixing the bugs, having another problem raised…
What I did in these six weeks was just repeating the above steps. However, the more questions raised and been asked, the more I learned and understood.
In this research project, we focused on an additive model consisting of a Fourier series and an autoregressive model, to model and simulate the seasonal and stochastic variations of the climate time series.
This approach is simple and flexible. There is no complicated algorithm involved, and it does not require any other year of data, sheet of data or data from neighbouring stations. As long as there is enough complete continuous piece of data in the data set for us to model the data characteristics of the variable, then we are able to fill the missing values of the same variable at whatever places in the data set.
I would say this research experience has been undoubtedly the most exciting event that happened this summer vacation. So what does the future hold? More modelling and more simulations on time series.
Hanyi Wang
University of South Australia
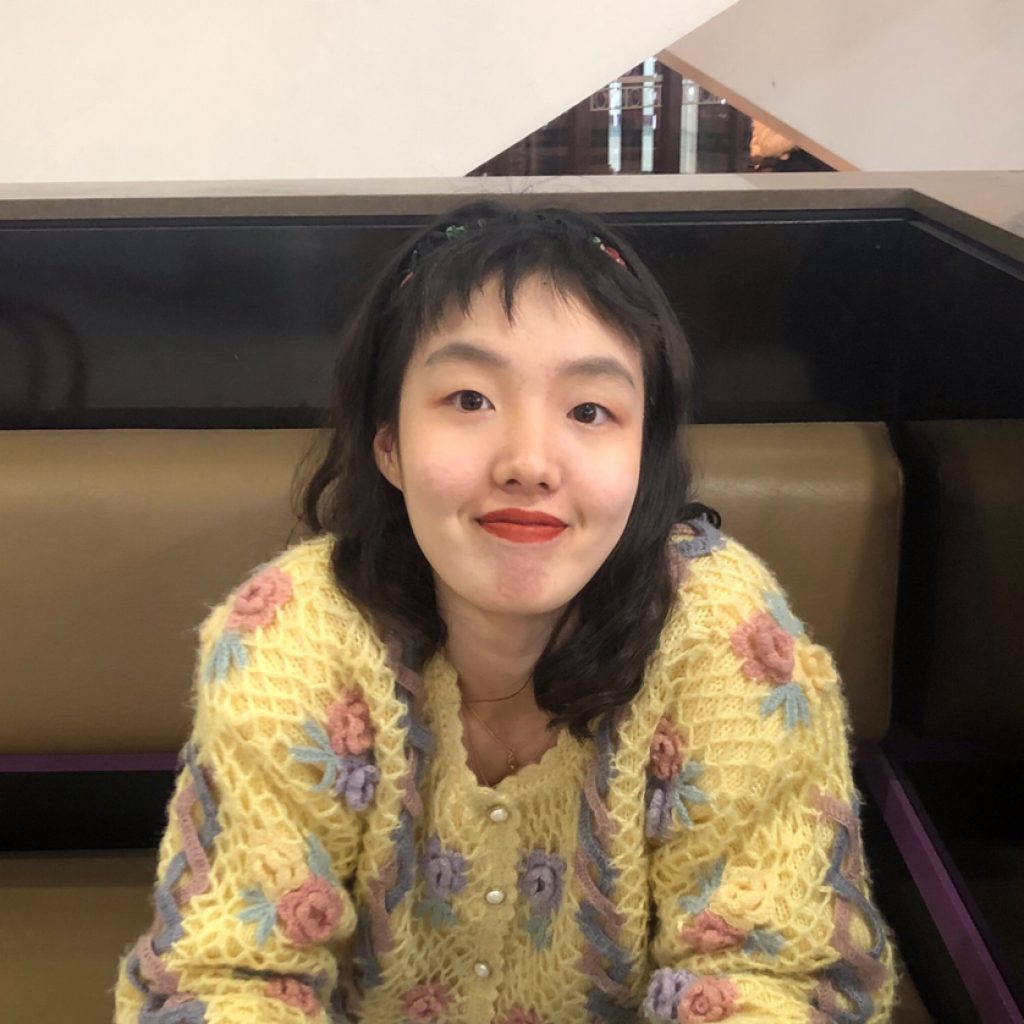