When you are looking at something, what you are seeing is not what you are getting. Allow me to explain this seemingly bewildering statement. When photons of light reflecting off an object are absorbed by the retinas in your eyes, they are just packets of sensory information that your brain receives. The brain has yet to interpret their meaning, which is what really needs to happen for you to recognise, say, the face of a loved one. To complicate things, sensory information can be noisy or/and ambiguous – think of the last time you were tricked by an illusion. So how does the brain interpret all this uncertain information to make rational decisions? Well, one powerful framework to think about it is good old fashioned Bayes theory.
Bayes theorem is expressed by the following formula:
‘Posterior’ = ‘Prior’ x ‘Likelihood’ x a constant
For the sake of simplification, we can ignore the constant term in this discussion and relax the equality to proportionality, so we can express the above as:
‘Posterior’ is proportional to ‘Prior’ x ‘Likelihood’.
Conceptually, the ‘prior’ can be thought of as pre-existing knowledge or expectations from accumulated past experiences that influences how the brain will interpret sensory information in the present.
Next, the ‘likelihood’ or sensory likelihood, as it applies to brains, represents the brain’s assessment of how well present sensory input matches its expectations or prior beliefs; you can think about it as a kind of sensory ‘evidence’.
Now, according to Bayes we can multiply the prior and the likelihood to get the posterior. The posterior represents brains combining prior expectations with sensory evidence to get a fuller, more updated picture of what the real world is.
My AMSI SRS topic delves into an application of Bayes theory to a dot motion direction estimation task. The objective of the research is to figure out if there is evidence to support the notion that our brains are performing Bayes theory to make estimates about the dot motion, or there are some other mathematical models that better fit the data. As it turns out, whilst the Bayes approach would be mathematically optimal, the data seems to support other simpler approaches that don’t necessarily require the same level of computations to be performed in the brain.
I’m fascinated by the power of statistical modelling to explain the world around us and hope to further my research of the human brain in the future using an increasingly expanding modelling toolkit. This AMSI summer research experience is my first step in that direction, and I am truly appreciative of the opportunity.
Chih Yuan (Yuan) Chan
University of New England
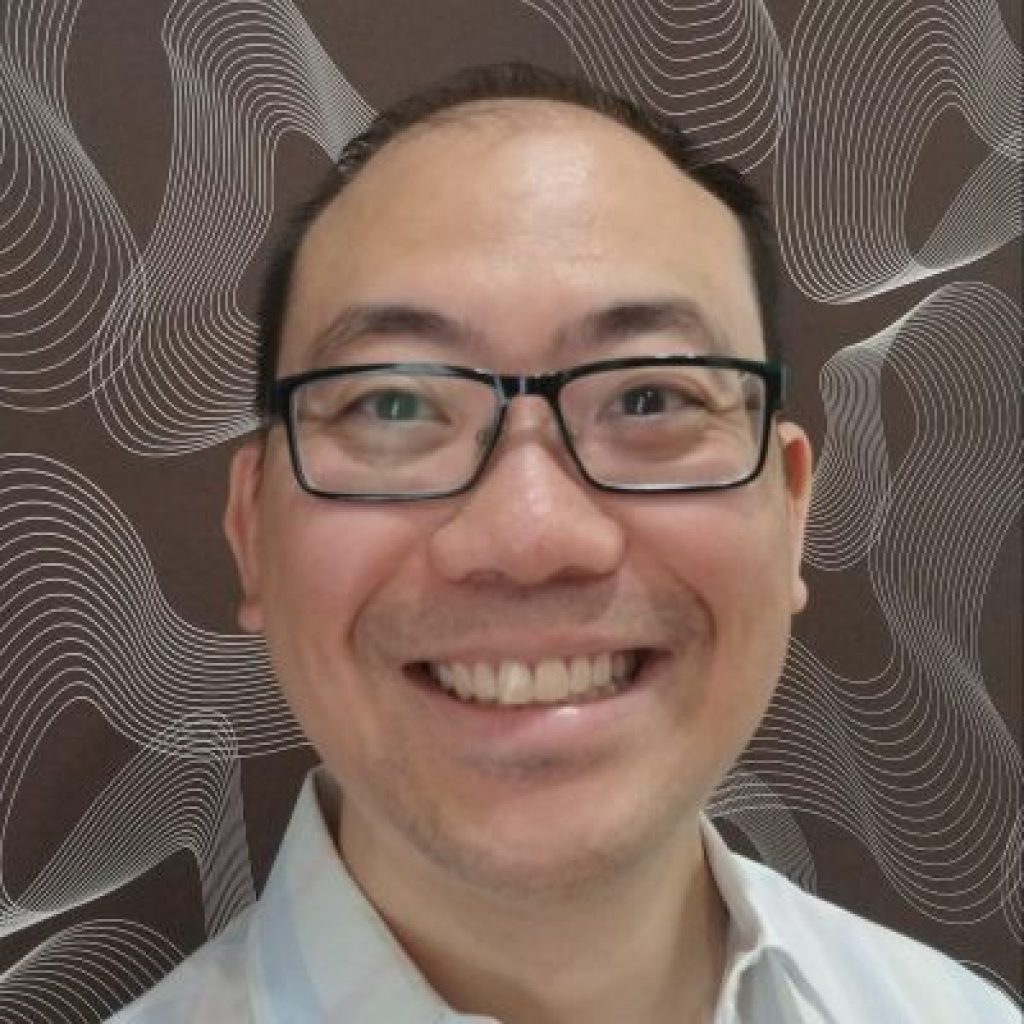