Abstract
Over the Summer Holidays, I investigated pricing models for a specific style of option called American options. I was first introduced to options through my Actuarial Studies degree’s finance course, although we only learnt about basic pricing models for the much simpler European option. American options have inspired many researchers to analyse them through various mathematical lenses such stochastic calculus, machine learning, even needing to borrow ideas from physics. So why does a seemingly simple financial instrument require such an elaborate toolkit?
In general, an option is a contract that allows the holder the option, with no obligation, to transact some other asset at an agreed price and date(s). A call option allows the holder to buy the asset at the agreed strike price, while a put option allows the holder to sell at strike.
Aristotle recorded the first option-like agreement in the mid-4th century BC about a man who could predict olive harvests by studying the stars. The man paid olive press owners to use them at a predetermined date (when he predicted the harvest to be plentiful). If the harvest was poor, then he would not use the press and only forfeit the initial fee; but his prediction came true, and he exercised his ownership right to profit handsomely. In essence, he exercised an early form of call option.
In a modern setting, options are typically listed on assets like stocks and commodities. Investors can combine these into more sophisticated strategies, for example, an option straddle that enables profit in both movement directions. Options that are allowed to be exercised at one agreed time (expiry) are ‘European’, while those that can be exercised any time before expiry are ‘American’, thus, a lot more complicated. There are also ‘exotic’ options, my favourites being compound options (options on the options) and game options (allows the seller to cancel the option early).
The difficulty of pricing options arises from a combination of complicating factors. The randomness of the underlying asset movement, non-linear payoff structures, path dependency and the influences of market psychology and friction to name a few. The most widely known pricing model is the the Black-Scholes equation for European options, and assumes asset prices move in a Geometric Brownian Motion which has roots in physics. However, older models like this make a wealth of simplifying assumptions that are certainly violated in real markets, such as the non-arbitrage principle. With advancements in computer science and machine learning, modern approaches have begun to favour data-driven models that rely less on strict assumptions and more on real-world observations. The model presented in my report merges a diverse range of aspects from recent literature on the pricing problem, and then compares our slightly modified implementation to these numerical results. One of the models was shown to reduce the variance of value estimates which could reduce issues in existing data-driven models such as overfitting.
Billy Bourdaniotis
The University of New South Wales
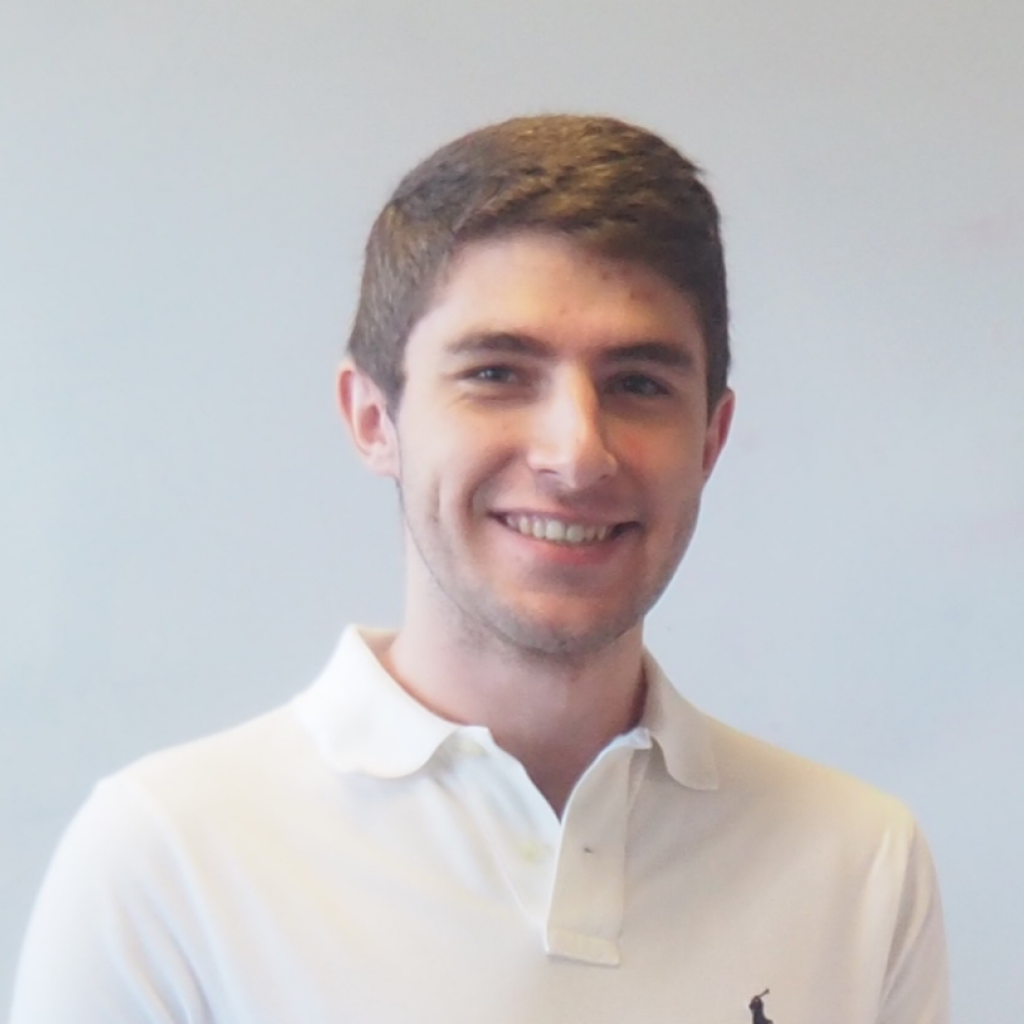