Abstract:
Mathematical models often have unknown parameters which can be determined by fitting them to data using Bayesian inference methods. By estimating these parameters, we are able to gain a greater understanding of the true data generating parameters for the model. Unfortunately, the computational requirements to accurately approximate the posterior can be immense and time consuming. Alternatives such as approximate likelihood techniques are much faster but much less accurate with existing calibration methods not being flexible enough to account for the complexity of some systems. Therefore, we propose a more flexible calibration technique, that maintains quick inference times as well as accurate inference.
Blog:
As we investigate complex mathematical models from realistic systems, there is an increasing need to understand how these systems behave. One way to do this is to estimate the unknown parameters of the system based on collected data.
To estimate these unknown parameters, scientists tend to use Bayesian inference. In Bayesian statistics we are interested in the posterior distribution, determined by prior knowledge of the parameters with information from the data that is summarised in a likelihood function. With this posterior, we are able to gain some understanding of the parameters that created the model. However, more complex models may have computationally infeasible intractable likelihoods.
To handle these intractable likelihoods, we often use alternative methods. One such method is the likelihood-free approximate Bayesian inference, which fits the model to the data using only simulations of the proposed model instead of evaluating the likelihood function. However, this requires tens of thousands of model simulations, resulting in computationally burdensome and slow inference. An alternative would be to use a surrogate likelihood instead of the intractable likelihood. This method is much quicker to evaluate but, the resulting approximate posterior may not be close to the desired posterior.
Scientists often use calibration techniques to improve the accuracy of approximate posteriors. One such technique is Bayesian Score Calibration (BSC), a calibration technique applied to the approximate posterior that uses a surrogate likelihood function. BSC optimises a scoring rule and simulates the model from in an attempt to learn a transformation to improve the approximate likelihoods accuracy. This method requires only hundreds of model simulations, making it much quicker than approximate Bayesian inference whilst also improving the accuracy of the approximate posteriors. However, this method does assume that bias introduced in the surrogate likelihood is constant across the parameter space, a fact that is not applicable to all surrogate likelihoods. Therefore, the resulting calibrated posterior may still be inaccurate.
As a result, an alternate calibration method is required to generate more flexible transformations and accurate calibration of posterior approximations. We propose the sequential Bayesian score calibration (SBSC) methods, an extension of BSC that sequentially applies transformations to the adjusted posterior to create a more flexible transformation.
Through SBSC, we are able to generate more flexible transformations to create a calibrated posterior that more accurately reflects the true data generating parameters of the system. As seen in the figure below, the posterior of 5 iterations of SBSC is able to represent the true data generating parameters than both the surrogate likelihood and the BSC. Furthermore, SBSC (16 minutes) was able to generate a posterior about 15 times faster than the exact Bayesian inference method (4 hours). Therefore, the SBSC method is able to produce an accurate posterior quickly for complex mathematical models.
Plot of posteriors of simulation-based inference posterior (approximate Bayesian inference) in black, PAL (surrogate likelihood), BSC adjusted posterior and 5th iteration of SBSC
Jack Fewtrell
Queensland University of Technology
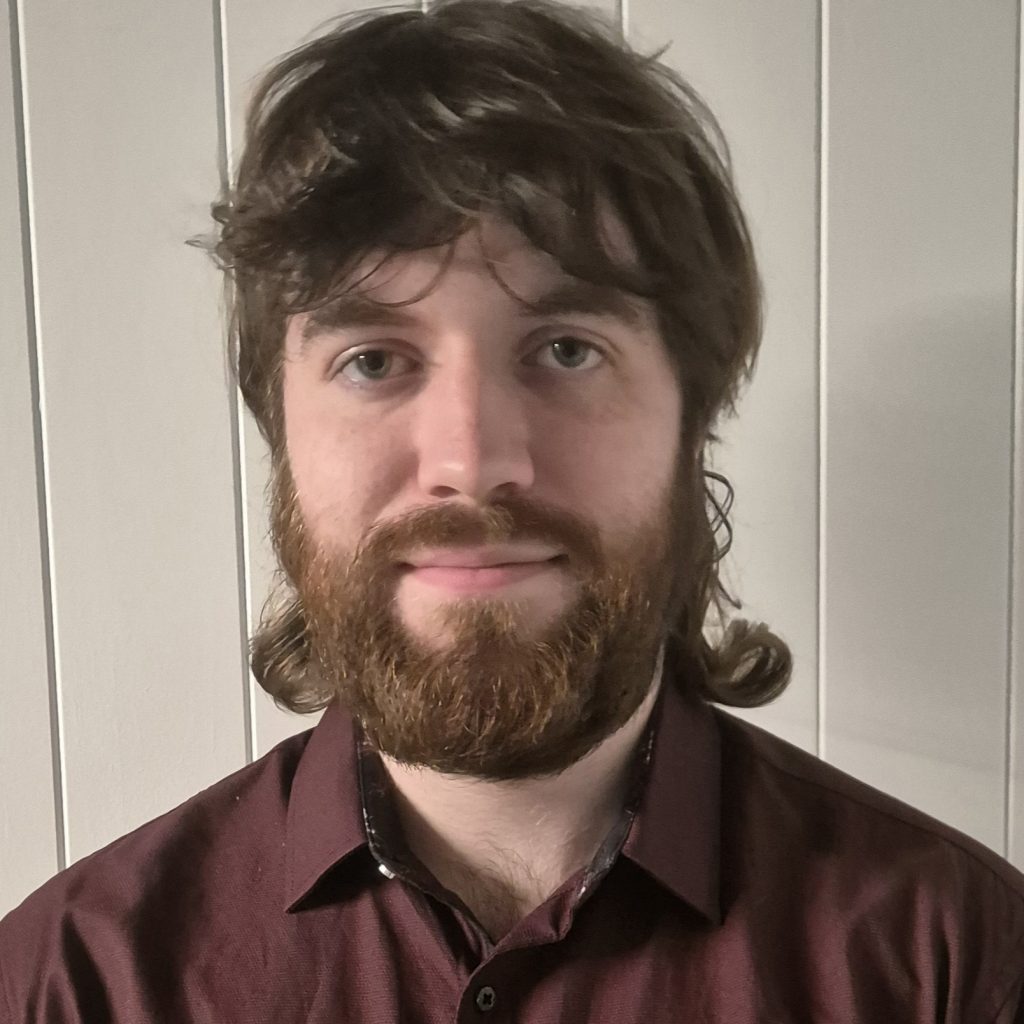