Abstract:
Cell Type Deconvolution is the process of uncovering cell type proportions in a biological tissue. Although it does not sound like it, but it is actually a statistical problem to resolve. The most interesting thing about it is that a particular method uses Latent Dirichlet Allocation, which was used in Natural Language Processing, a completely different area to Biology. Many fascinating results are obtained, which have led to breakthroughs in the field of genetics.
Blog:
For many people, Mathematics remains a profound yet mysterious area that seemingly lacks practical connection to our daily lives. However, this perception is incorrect! In statistics, a crucial branch of mathematics, many endeavours demonstrate close relationships with other fields such as Computer Science, Biology, and Engineering. Cell type deconvolution serves as a prime example, showcasing remarkable results achievable when statistical methods are applied to biological data.
Naturally, several questions arise:
- What is Cell Type Deconvolution?
- Which statistical methods are utilized?
- What remarkable outcomes does this offer biologists?
Addressing the first question is straightforward. Within a biological tissue sample, different sections in the spatial structure often correspond to distinct cell types. Through the advancement of Spatial Transcriptomics (ST) technology, gene expression data can be collected for various spots on the tissue sample. The objective is to utilize this spatial data to identify cell types for each spot. However, cell types do not directly manifest in the spatial data, prompting researchers to typically incorporate a second dataset – a reference matrix.
The purpose of this reference matrix is to inform the model about the likelihood of each gene occurring for each cell type. However, as there’s no free lunch, this reference matrix may lack accuracy. How can we leverage all available information while accounting for potential accuracy issues? Perhaps by employing it as a prior!
This leads to the second question, where the underlying statistical method is Latent Dirichlet Allocation. Surprisingly, it was initially a Natural Language Processing technique for discerning topic distributions in a document of texts. In our model, each spot is assumed to contain numerous molecules, with each molecule attributed to a certain cell type (which, unfortunately, we don’t directly observe in the spatial data) and representing a specific gene. Employing this model, we can integrate the reference into the final step, where the likelihood for the occurrence of each gene for each cell type is provided by the reference matrix.
So, what are the outcomes? Primarily, cell types can be identified for different parts of the sample, which is crucial for several reasons. Many downstream analyses can be conducted based on the deconvolution results (such as interpreting identified transcript programs); furthermore, it may lead to disease discovery and drug development.
Of course, much work remains, but the most significant aspect is not to confine mathematics to an incomprehensible study of numbers and symbols. Instead, we must recognize how it has and will continue to assist us in overcoming challenges in our world.
Yichen Jiang
The University of Melbourne
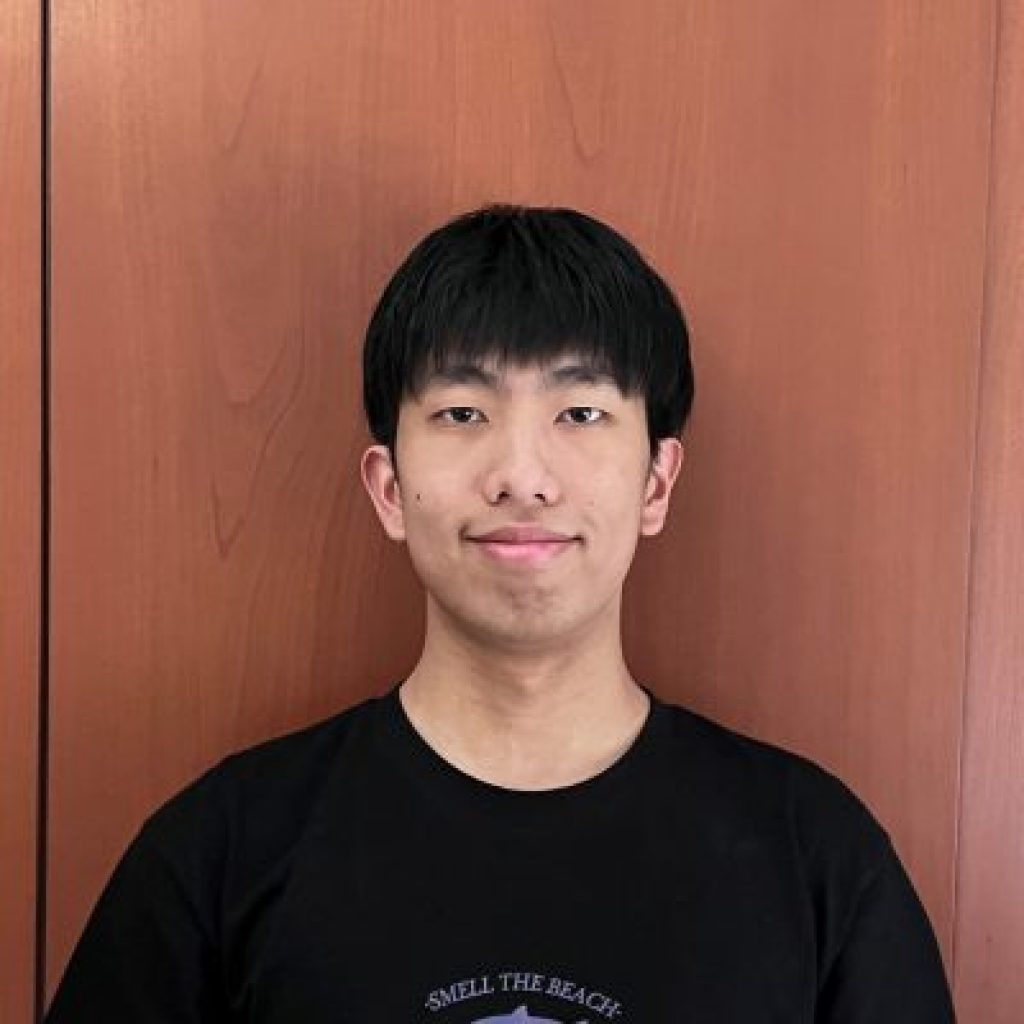