Australia faces unprecedented challenges over the coming decade. We need to fight off COVID-19 and rebuild the economy. We need to build climate resilience and address the enormity of Australia’s greenhouse gas emissions. And, we will need to confront the threat of AI and automation to full employment and workers’ rights.
These are thorny problems that require multilateral cooperation and a whole lot of innovative public policy. In this post, I talk about how I believe that mathematics and statistics underpin the next generation of policy.
We have plenty of reason to be optimistic about Australia’s capacity for data-driven policy. Why? Just look at the incredible things underway. There are so many cases of incredible statistical solutions to thorny policy problems, including CSIRO researchers using a simple game to identify behavioural patterns indicative of mental health problems, and researchers from Melbourne Uni using new predictive algorithms to detect worker exploitation and wage theft.
The public sector is also rapidly innovating, particularly on issues of data privacy and availability. The emergence of securely linked data projects, such as MADIP for citizens and BLADE for businesses, is revolutionising the tools for evidence-driven policy. High quality and reliable data mean policy impacts can be better conceived, predicted, and evaluated.
Among others, there are two basic preconditions to taking the data opportunity in policy. We should build the ecosystem and reject our biases.
Build the ecosystem
Building the ecosystem means that we need to invest broadly to build capacity. We need to create school curriculums that teach data literacy and encourage young people to enter high-growth industries. It also means that we should fund basic research into mathematics and physics, even when it’s hard to see or understand the impact.
Research into basic sciences opens up opportunities we could never have imagined. The computational power of quantum algorithms promises to open up new unthinkable applications such as rapid pharmaceutical discovery and new natural language processing models. This is impossible without the fundamental research into mathematics and physics: especially when that research looks like it has no direct applications.
Reject our biases
We also need to reflect and consider the biases we bring to our use of data. By the nature of a crisis, we may only get one chance to get our response right – as was the case with COVID-19. We should approach a problem with fresh eyes instead of presupposing a solution. In the case of data, rejecting our biases has two meanings: first, we should use the data to draw out new insight, and not hunt for evidence to support our current beliefs (p-hacking). Second, we should consider the biases embedded in data. Are we training models on racist, sexist, or otherwise biased data that will produce sub-optimal outputs?
If we can reject our biases to draw insight from data and build an ecosystem that unlocks emerging potential, then we are well on our way to tackling the next generation of challenges.
Luke Thomas
The University of Western Australia
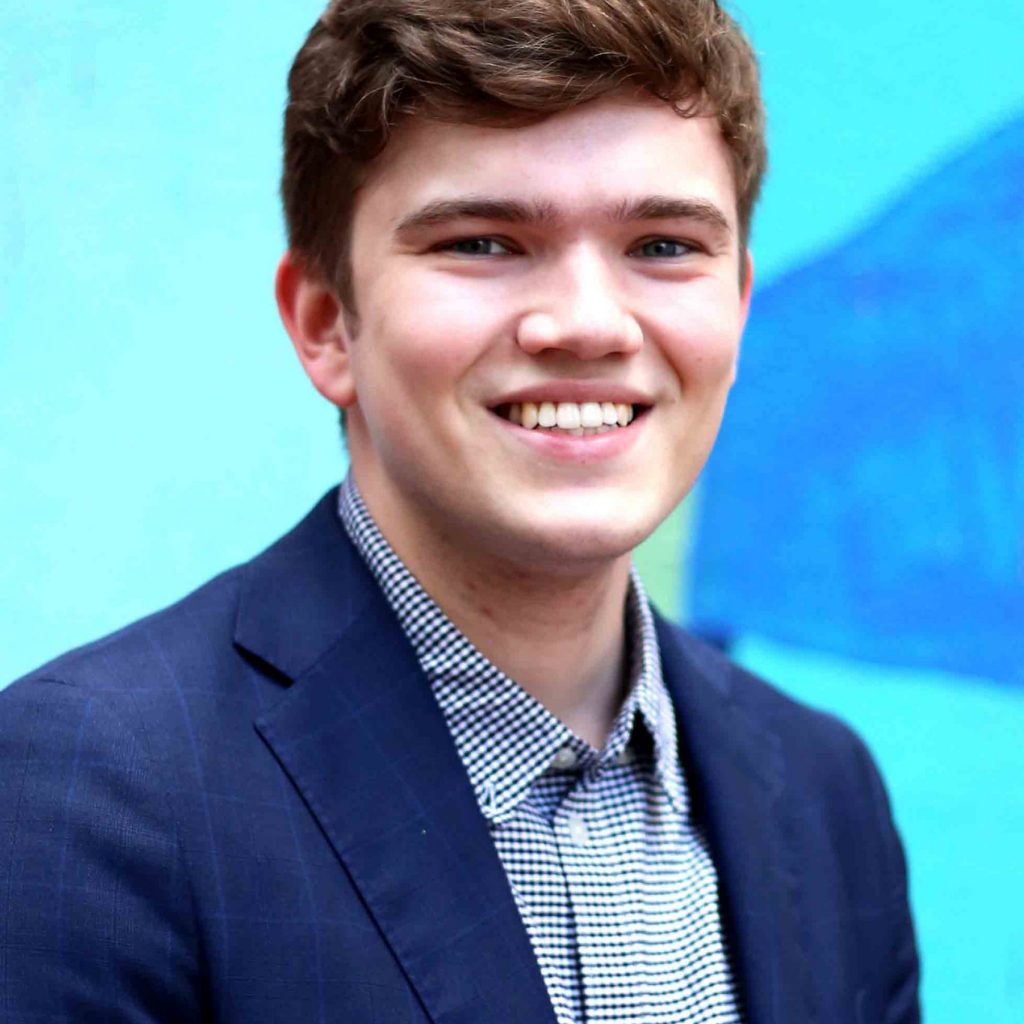