There has been a long debate between Bayesian statistics and frequentist statistics. What is the difference between them? Should I use Bayesian statistical methods for data processing? Is one method better than the other? This blog will introduce the basic ideas of Bayesian statistics and its difference between frequentist statistics using some simple examples.
The bedstone of Bayesian statistics is the Bayes Theorem, which describes the probability of the occurrence of an event based on historical knowledge and observations.
Classical frequentists tend to assume that this world is only one way – everything is fixed and has a true underlying parameter. However, a Bayesian partisan might argue that this idea is ridiculous!
Instead of the parameter being fixed, Bayesian posits that it first follows a specific distribution – known as the prior distribution, that is, before doing any experiment or measurement, they assign a belief state to it. This might come from the historical evidence, known results… The second step is to run the experiment and collect some data to calculate the probability of different values of the parameter interested GIVEN the observed data – known as the posterior distribution.
Let’s further compare the difference between how these two factions express their uncertainty after an experiment. For frequentists, they use a “confidence interval” to stand for a range of values to include the true parameter value with some probability, usually 95%. The interpretation is a little bit tricky – if you repeat this experiment 100 times, you would expect at least 95 of the resulting confidence intervals will include the true value of the parameter. What about the other 5? Well, one case is they are slightly OR completely non-sense!
Back to the perspective of Bayesian. After we got the new probability distribution – the probability of different values of the parameter given the observed data and the prior – the posterior, we can summarize the information of an unobserved value based on the posterior distribution. The interpretation of credible interval is much simpler than confidence interval! Let’s say we have a 95% credible interval, then it is just the central portion of the posterior distribution that contains 95% of the values.
Therefore, Bayesian partisans might argue that “Allowing 5 experiments to be completely non-sense is ridiculous! You only care about the majority of experiments!”
And frequentist might punch back the credible interval like “I want a method that works for any possible value, I only care about the single true value it does have. And your method is largely dependent on the prior!”
Yuxin Yan
The University of Melbourne
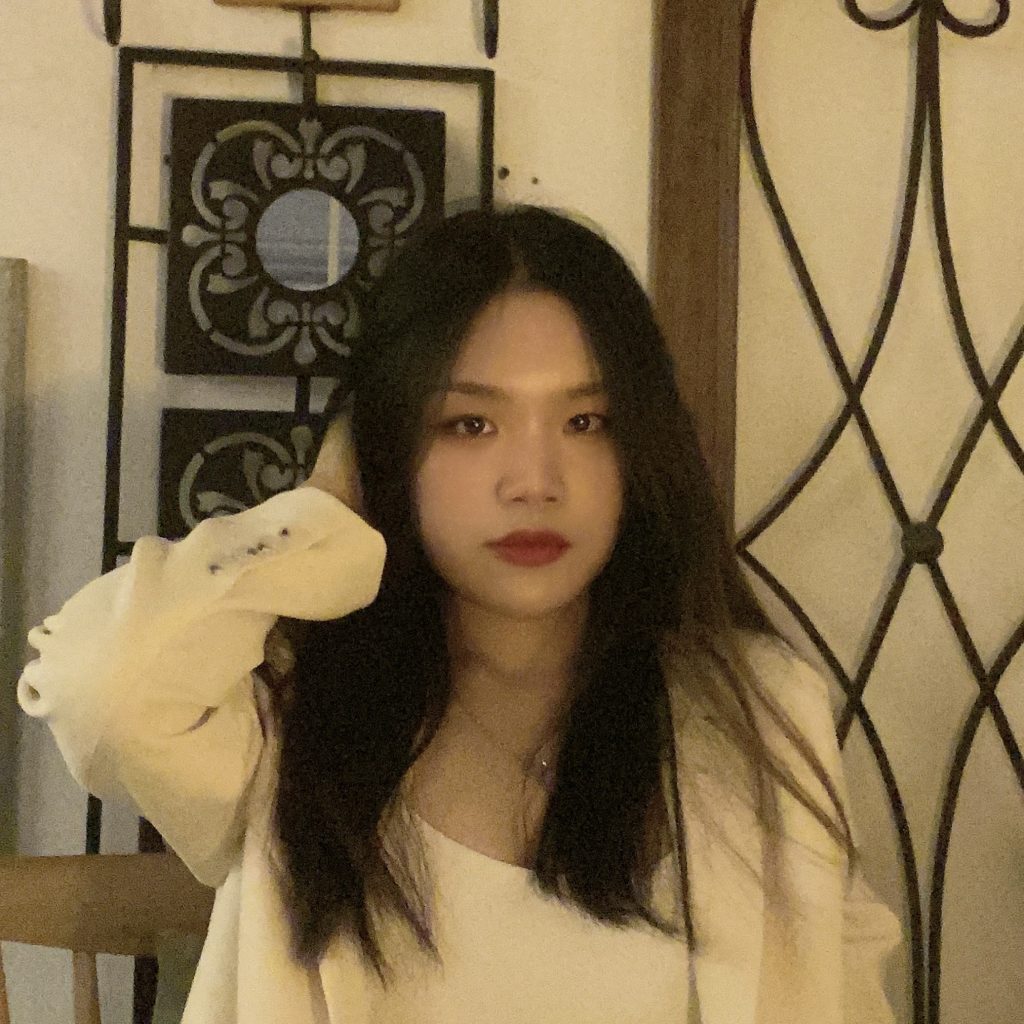